Enhancing Markerless Motion Capture System Using Multi-View Neural Rendering and Temporal Consistency Models.
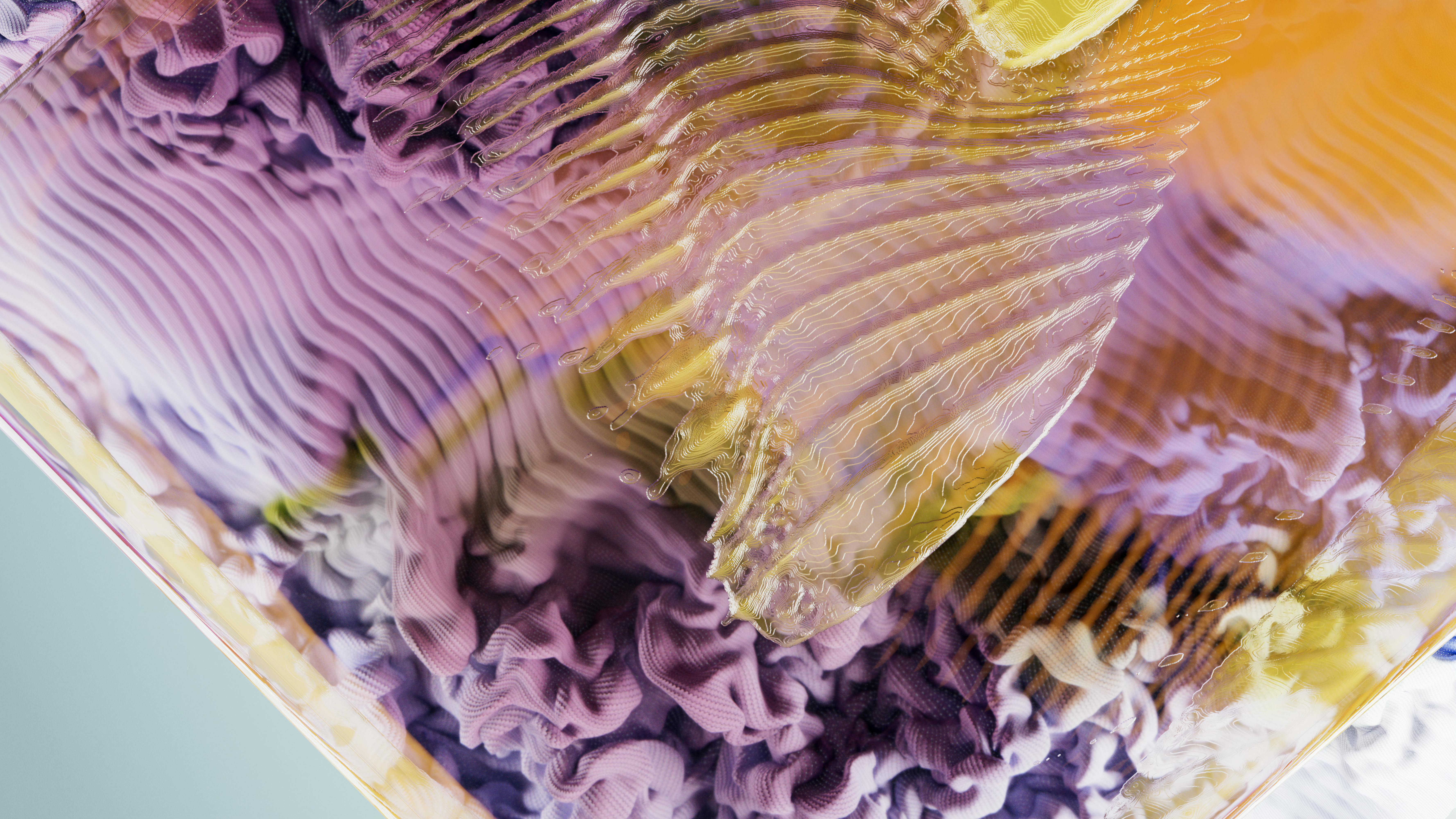
Markerless motion capture (MoCap) systems have revolutionized industries such as gaming, film production, healthcare, and sports by eliminating the need for intrusive markers and suits. However, challenges such as occlusions, inaccurate joint tracking, and temporal inconsistencies persist. Recent advancements in multi-view neural rendering and temporal consistency models present promising solutions. This article explores how these technologies can enhance markerless MoCap systems by improving accuracy, robustness, and realism.
Marker-based motion capture systems rely on reflective markers attached to the subject, tracked by an array of cameras. While effective, these systems are expensive, labor-intensive, and intrusive. Markerless MoCap systems, which use computer vision and machine learning, aim to overcome these limitations by analyzing video data directly. However, challenges such as occlusions, ambiguities in 3D reconstruction, and temporal noise hinder their widespread adoption. The integration of multi-view neural rendering and temporal consistency models offers a pathway to mitigate these issues. Multi-view neural rendering leverages multiple perspectives to create detailed and coherent 3D reconstructions, while temporal consistency models ensure smooth transitions over time, addressing jitter and noise in motion data.
Multi-view neural rendering is a computational technique that synthesizes 3D models from multiple camera perspectives using neural networks. Key innovations in this area include Neural Radiance Fields (NeRF), which employ volumetric rendering techniques combined with neural networks to generate high-quality 3D reconstructions from 2D images. By encoding spatial information and color across multiple views, NeRF creates detailed and accurate reconstructions, even in challenging scenarios involving occlusions or sparse camera setups. Cross-view consistency systems, like PixelSynth and Neural Volumes, ensure that reconstructed models are consistent across multiple views, reducing artifacts and improving the overall fidelity of motion capture. These technologies enhance markerless MoCap by enabling robust 3D reconstructions, particularly in scenarios with limited camera coverage or complex interactions.
Temporal inconsistencies, such as jittery or disjointed motion, are common challenges in markerless MoCap. Temporal consistency models address these issues by utilizing Recurrent Neural Networks (RNNs), such as Long Short-Term Memory (LSTM) networks, which are effective for modeling sequential data. They can smooth out noise in motion trajectories by learning temporal patterns. Temporal smoothing networks, including Graph-based and convolutional models like Temporal Convolutional Networks (TCNs), analyze motion data over extended time windows, ensuring realistic transitions and reducing artifacts. Physics-informed models incorporate physical constraints into neural networks, ensuring that reconstructed motions adhere to realistic biomechanical principles, enhancing the plausibility of captured movements.
The synergy between multi-view neural rendering and temporal consistency models enhances markerless MoCap in several ways. Multi-view neural rendering captures complex scenes and occluded joints accurately, while temporal models refine these reconstructions over time. Temporal consistency models eliminate jitter and disjointed transitions in motion trajectories, producing smooth and realistic outputs. Combining these approaches allows for robust markerless MoCap systems that can operate in diverse environments, from professional studios to outdoor settings.
A notable player in advancing the frontiers of markerless MoCap technology is Move AI. By leveraging cutting-edge research and proprietary algorithms, Move AI has addressed many of the challenges traditionally faced in this field. Their solutions incorporate multi-view neural rendering to achieve high-precision 3D reconstructions, even in scenarios with limited camera setups or complex subject interactions. Additionally, Move AI’s temporal consistency frameworks ensure smooth, artifact-free motion trajectories, enabling seamless integration into professional workflows. With a focus on scalability and accessibility, Move AI is paving the way for markerless MoCap systems to be adopted widely across industries, from gaming and entertainment to healthcare and sports.
Enhanced markerless MoCap systems have broad applications, including realistic character animations for films and video games, detailed biomechanical analysis of athletes’ movements for performance optimization, non-intrusive monitoring of patients for rehabilitation and physical therapy, and improved human-robot interaction through accurate motion tracking and prediction. While promising, integrating multi-view neural rendering and temporal consistency models presents challenges, such as high computational requirements, the need for large, high-quality datasets, and ensuring model generalization across diverse scenarios and environments.
Future research should focus on developing efficient models that can run on consumer-grade hardware, leveraging unsupervised and semi-supervised methods to reduce dependency on labeled data, and combining marker-based and markerless approaches for enhanced flexibility and accuracy. The integration of multi-view neural rendering and temporal consistency models represents a significant advancement in markerless motion capture technology. By addressing challenges such as occlusions, temporal noise, and scalability, these innovations promise to make MoCap systems more accurate, robust, and accessible across various industries. Continued research and development in this area will undoubtedly unlock new possibilities and applications for motion capture technology.